Self-healing Masterdata And (Planning Systems) Parameters
WHY (Problem)
Supply Chain Planning systems are only as accurate as their master data and parameters. Most of these are maintained manually by planners, which inevitably leads to human errors.
Planning IT landscapes typically consist of multiple systems, each requiring the maintenance of the same parameters (e.g., transit time, calendars, network) but using different logical definition. For example, systems like SAP APO/IBP, SAP ECC, OMP, and Maestro each require a different approach to configure 'site' calendars. This parallel manual maintenance results in planning attributes not being aligned across the end-to-end supply chain.
Planning parameters should reflect operating strategy choices or the physical reality and performance of the supply chain. The latter (e.g., transit times, production reliability, throughput) are rapidly changing, and planners often fail to update the systems with quality data.
Mistakes in master data and parameter maintenance, lack of alignment across systems, and failure to reflect supply chain reality are common reasons for inaccurate or suboptimal supply chain planning decisions, leading to service losses, increased costs, or excess inventory.
WHAT (Value)
Productivity
of planners' time saved, previously spent on master data and parameters maintenance, audits, and updates.
accuracy of master data and parameters.
synchronization of parameters across systems.
adjustment of parameters to reflect the physical supply chain reality.
Note: Above leads to 1-10% better supply chain planning results in terms of Customer Service and keeping Inventory within limits.
Time to Value
Complexity:
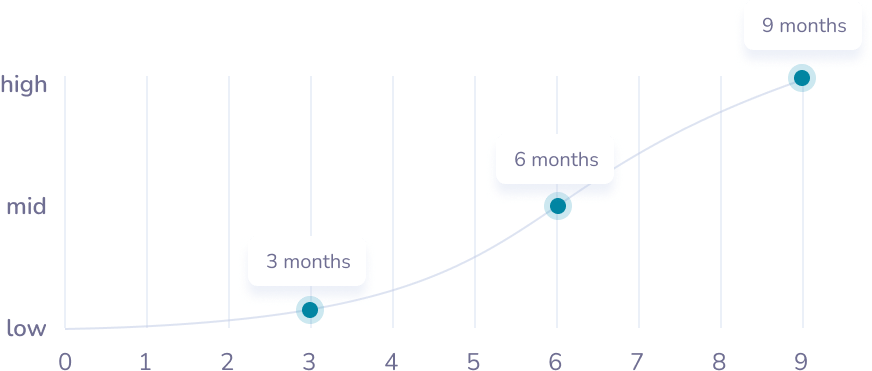
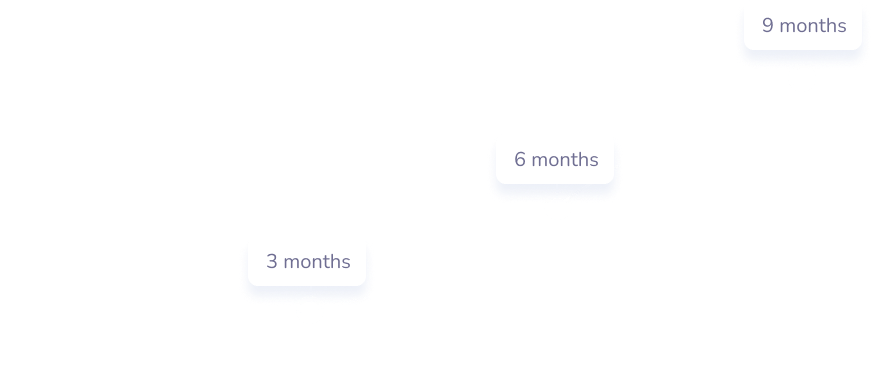
Decision quality
98-100% accuracy of master data and parameters.
100% synchronization of parameters across systems.
Daily/weekly adjustment of parameters to reflect the physical supply chain reality.
HOW (Solution)
(usually mistakes), determine correct maintenance patterns, synchronize parameters
across systems, and adjust to supply chain reality (represented by actual operational data). Our algorithms effectively combine business rules with clustering machine learning algorithms. They encapsulate key parameter definitions for the most popular APS and are designed to work on top of it to ensure always-correct parameters.